Key Takeaways
- Machine Learning Dominance: Google’s RankBrain signifies a pivotal shift in SEO, harnessing the power of machine learning to interpret user intent and adapt to evolving search trends.
- User-Centric Strategies: Aligning content creation with user intent is paramount. User engagement metrics, semantic relevance, and mobile optimization are pivotal for success in the RankBrain era.
- Future-Proof SEO: Embrace the future of SEO by staying updated on algorithmic changes, leveraging tools like Google Analytics and SEMrush, and understanding the evolving landscape of machine learning, ensuring enduring success in search rankings.
In the dynamic realm of search engine optimization (SEO), staying ahead of the curve is paramount to achieving online visibility and success.
Google, the unrivaled titan of the digital landscape, continually refines its algorithms to provide users with the most relevant and high-quality search results.
One such groundbreaking development that has significantly influenced SEO strategies is Google’s RankBrain algorithm, a revolutionary leap into the domain of machine learning.
In this comprehensive exploration, we delve into the intricacies of Google’s RankBrain Algorithm, unraveling the complex tapestry of machine learning within the SEO ecosystem.
As search engines evolve beyond traditional ranking factors, understanding the mechanics of RankBrain becomes not just advantageous but imperative for digital marketers, content creators, and businesses seeking to optimize their online presence.
Unveiling the Enigma
Google’s RankBrain, introduced in 2015, marked a paradigm shift in the world of search algorithms.
Unlike its predecessors, RankBrain incorporates machine learning to comprehend the nuances of user queries and web content, allowing it to deliver more nuanced and contextually relevant search results.
As we embark on this journey of comprehension, we’ll demystify the core principles that underpin RankBrain’s functionality.
The Evolutionary Arc
To comprehend RankBrain fully, one must first grasp the evolutionary trajectory of Google’s algorithms. From the rudimentary algorithms of yesteryears to the sophisticated machine learning models of today, the landscape has transformed drastically.
We’ll traverse this historical narrative, shedding light on the pivotal moments that led to the inception of RankBrain and its subsequent integration into the search engine giant’s ranking criteria.
Unraveling the Threads of RankBrain
At its essence, RankBrain is an artificial intelligence (AI) system that employs neural networks to interpret and learn patterns from vast amounts of data.
But what sets it apart?
How does it augment the traditional algorithms, and what role does machine learning play in this intricate dance of ranking signals?
These questions form the crux of our exploration as we navigate the inner workings of RankBrain.
A Primer on Machine Learning
Before delving into RankBrain’s specifics, a foundational understanding of machine learning is imperative.
We’ll embark on a journey into the realm of neural networks, algorithms, and the iterative learning process that defines machine learning. By establishing this baseline, we empower ourselves to decipher the symbiotic relationship between machine learning and SEO.
Neural Networks: The Cognitive Architecture
RankBrain’s prowess lies in its ability to mimic the cognitive functions of the human brain through neural networks.
These interconnected nodes process information, adapt to patterns and refine their understanding over time.
We’ll dissect the anatomy of these neural networks, unveiling the underlying brilliance that enables RankBrain to navigate the vast expanse of the internet and discern relevance with unprecedented accuracy.
Factors on RankBrain’s Canvas
As we peer into the algorithmic canvas of RankBrain, a multitude of factors come into play.
From user experience signals to content relevance, each element contributes to the algorithm’s ability to discern the best possible results.
We’ll illuminate these factors, providing actionable insights into crafting content and strategies that resonate with RankBrain’s discerning gaze.
Embark on this enlightening expedition as we unravel the enigma of RankBrain, demystifying machine learning in SEO to empower you with the knowledge needed to navigate the ever-evolving landscape of digital visibility.
Join us in deciphering the algorithms, exploring optimization strategies, and unlocking the untold potential of understanding Google’s RankBrain Algorithm.
As the digital horizon continues to expand, the key to success lies in adapting and thriving within the intricate dance of machine learning and SEO.
Before we venture further, we like to share who we are and our digital experiences.
About AppLabx
From developing a solid marketing plan to creating compelling content, optimizing for search engines, leveraging social media, and utilizing paid advertising, AppLabx offers a comprehensive suite of digital marketing services designed to drive growth and profitability for your business.
AppLabx is well known for helping companies and startups use SEO to drive web traffic to their websites and web apps.
At AppLabx, we understand that no two businesses are alike. That’s why we take a personalized approach to every project, working closely with our clients to understand their unique needs and goals, and developing customized strategies to help them achieve success.
If you need a digital consultation, then send in an inquiry here.
Google’s RankBrain Algorithm: Understanding Machine Learning in SEO
- The Basics of RankBrain
- How RankBrain Works
- Impact on SEO Strategies
- Optimizing for RankBrain
- Future Trends in Machine Learning and SEO
1. The Basics of RankBrain
Definition and Purpose
Defining RankBrain
At its core, RankBrain is a machine learning algorithm developed by Google to enhance the search engine’s ability to understand and interpret user queries.
Introduced in 2015, this sophisticated artificial intelligence system plays a pivotal role in determining the relevance of search results by learning from patterns and adapting to evolving search trends.
Purpose of RankBrain
RankBrain addresses the limitations of traditional algorithms by introducing an element of artificial intelligence into the search process.
Its primary purpose is to handle ambiguous or novel queries that traditional algorithms may struggle to interpret accurately.
By leveraging machine learning, RankBrain refines its understanding over time, continuously improving its ability to deliver more contextually relevant search results.
Evolution of Google’s Algorithms
Historical Context
To appreciate RankBrain’s significance, we must trace the evolution of Google’s algorithms.
From the introduction of PageRank in the late 1990s to the incorporation of machine learning in the form of RankBrain, Google’s journey has been marked by a relentless pursuit of providing users with the most accurate and valuable information.
RankBrain in the Algorithmic Landscape
RankBrain represents a departure from static algorithms to dynamic, self-learning systems.
Traditional algorithms relied on predefined rules, whereas RankBrain, with its machine learning capabilities, can adapt to the ever-changing nature of user queries and content on the internet.
This evolution signifies Google’s commitment to enhancing the search experience through cutting-edge technology.
Role of RankBrain in Search Engine Ranking
Dynamic Query Interpretation
RankBrain’s key contribution lies in its ability to interpret and understand queries in a more nuanced manner.
For instance, if a user enters a query with ambiguous terms or misspellings, RankBrain can decipher the underlying intent and deliver results that align with what the user is seeking.
Adapting to User Behavior
Unlike static algorithms that may struggle to adapt to shifts in user behaviour, RankBrain analyzes user interactions with search results.
It takes into account metrics such as click-through rates and dwell time to evaluate the relevance and satisfaction of search results.
This adaptability ensures that the algorithm evolves with changing user preferences and trends.
Examples
Contextual Understanding
Consider a search query like “best budget smartphones for photography.”
While traditional algorithms might focus on exact keyword matches, RankBrain comprehends the user’s intent, emphasizing the importance of budget-friendly options specifically tailored for photography enthusiasts.

Improved Ambiguity Handling
For queries like “home remedies for aching joints,” RankBrain excels at understanding the user’s need for natural solutions to joint pain.
It goes beyond literal keyword matching, showcasing an ability to navigate and interpret the intricacies of natural language.
Impact on Search Results and User Experience
Enhanced Relevance
RankBrain’s machine learning capabilities contribute to a more refined ranking process, ensuring that search results align closely with user intent. This emphasis on relevance enhances the overall quality of search results, elevating the user experience.
Personalization and Localization
RankBrain contributes to the personalization of search results based on individual user behaviour.
For instance, a user in New York searching for “best coffee shops” may receive different results than someone in San Francisco. This localization adds a layer of customization, tailoring results to specific geographical contexts.
Impact on Search Queries
A study revealed that RankBrain is one of the top three ranking factors, highlighting its significant influence on search results.
With over 15% of entirely new search queries, its learning capabilities ensure that Google can provide relevant results even for queries it has never seen before, emphasizing the pervasive role of RankBrain in the search ecosystem.
User Satisfaction Metrics
According to Google, RankBrain is highly successful at improving user satisfaction.
In a statement, they mentioned that RankBrain is the third-most important ranking factor and has resulted in significantly better search results, enhancing the overall search experience for users.
In unravelling the basics of RankBrain, we’ve explored its definition, purpose, evolution, and pivotal role in shaping search engine rankings.
As we delve deeper into its mechanics, we’ll navigate the intricacies of how RankBrain works, shedding light on the neural networks and factors that drive its decision-making process.
Join us on this enlightening journey into the heart of machine learning within the SEO landscape.
2. How RankBrain Works
Introduction to Machine Learning
Machine Learning in the Context of SEO
Understanding how RankBrain works necessitates a grasp of the broader concept of machine learning.
In the SEO landscape, machine learning empowers algorithms like RankBrain to analyze vast amounts of data, recognize patterns, and autonomously adapt to the evolving nature of user queries and content.
RankBrain’s Position in Google’s Algorithm
RankBrain operates as part of Google’s Hummingbird algorithm, emphasizing the importance of understanding the broader algorithmic framework.
This integration allows RankBrain to contribute its machine-learning capabilities to the overall process of ranking web pages.
Neural Networks and RankBrain’s Learning Process
Neural Networks: The Cognitive Engine
At the core of RankBrain’s learning process are neural networks, computational models inspired by the human brain.
These interconnected nodes, or neurons, process information and learn from patterns.
This neural architecture enables RankBrain to discern context, relevance, and user intent with a level of sophistication that traditional algorithms may struggle to achieve.
Continuous Learning Iterations
RankBrain doesn’t rely on static rules; instead, it engages in continuous learning.
When faced with new or ambiguous queries, it adapts its understanding by drawing insights from historical data and user interactions.
This iterative learning process positions RankBrain as a dynamic and evolving force within Google’s ranking algorithm.
Factors Considered by RankBrain in Ranking Websites
User Engagement Signals
RankBrain places significant emphasis on user engagement signals, including click-through rates (CTR) and dwell time.
For instance, if a user clicks on a search result and spends considerable time on the page, RankBrain interprets this as a positive signal, indicating the relevance and satisfaction of the content.
Content Relevance and Quality
The algorithm assesses content relevance by evaluating how well it aligns with the user’s intent.
Quality, measured through factors like comprehensive information, readability, and multimedia elements, plays a crucial role.
in addition, high-quality content is correlated with higher search engine rankings.
Semantic Search and Contextual Understanding
RankBrain excels in semantic search, understanding the context and meaning behind words.
For instance, a search for “Apple” may be interpreted differently based on context—whether the user is interested in the tech company or the fruit. This nuanced understanding enables RankBrain to deliver more accurate results.
Examples
Ambiguous Queries
Consider a query like “Java.”
While it could refer to the programming language or the beverage, RankBrain, through its contextual understanding, distinguishes between these meanings.
This ability to navigate ambiguity showcases RankBrain’s capacity to provide relevant results for diverse user intents.
Evolving Search Trends
As user behaviour and search trends evolve, RankBrain adapts.
For instance, the rise of voice search has led to more conversational queries. RankBrain’s machine learning enables it to understand and respond to these shifts, ensuring that search results remain relevant in the face of changing user interactions.
Google is constantly working on improving RankBrain and making it more effective in understanding user queries.
This commitment to ongoing refinement reinforces the significance of RankBrain in the search engine giant’s strategy.
In comprehending how RankBrain works, we’ve explored the foundations of machine learning, the neural networks driving RankBrain’s cognitive capabilities, and the multifaceted factors it considers in ranking websites.
As we journey further into the impact of RankBrain on SEO strategies, we’ll uncover the evolving landscape of optimization techniques tailored to meet the demands of this dynamic algorithm.
Join us in unravelling the intricacies of machine learning in SEO, unlocking the keys to navigating the ever-changing terrain of search engine rankings.
3. Impact on SEO Strategies
Traditional SEO vs. RankBrain SEO
Shifting Paradigms
The advent of RankBrain has necessitated a paradigm shift in SEO strategies.
Traditional SEO, focused on static algorithms and keyword optimization, is now complemented by a dynamic approach that acknowledges the influence of machine learning on search rankings.
Keyword Intent and Semantic Relevance
RankBrain’s emphasis on user intent over exact keyword matches prompts a reevaluation of keyword strategies.
SEO practitioners now prioritize creating content that aligns with the semantic context of user queries, ensuring relevance and a better chance of ranking high on search engine results pages (SERPs).
Adapting to Machine Learning for Better Rankings
Content Depth and Quality
The impact of RankBrain underscores the importance of comprehensive, high-quality content.
Longer, informative content tends to perform better, meeting the criteria set by machine learning algorithms for relevance and user satisfaction.
User Engagement Metrics
RankBrain places a premium on user engagement metrics, emphasizing the need for content that captivates and satisfies users.
Click-through rates (CTR) and bounce rates are pivotal in signalling to RankBrain that the content is resonating with the audience, as cited by Timothy J. Miller.
Mobile Optimization
Given the increasing prevalence of mobile searches, optimizing for mobile has become imperative.
Google’s mobile-first indexing, combined with RankBrain’s adaptability to different devices, makes mobile optimization a crucial component of SEO strategies.
Google’s PageSpeed Insights tool is invaluable for assessing and enhancing mobile page performance.
Common Misconceptions About RankBrain
Oversimplification of Keywords
A common misconception is oversimplifying keywords and neglecting the importance of context.
RankBrain is designed to comprehend the intricacies of language, and SEO strategies should reflect this by addressing user intent through contextually rich content rather than relying solely on exact keyword matches.
Ignoring User Experience Signals
Some practitioners underestimate the significance of user experience signals in RankBrain’s decision-making process.
Metrics like dwell time, which measures the duration users spend on a webpage, and click-through rates contribute substantially to the algorithm’s understanding of content relevance and quality.
According to a report by the STAT Team, CTR and rank are causal.
As we proceed to unravel the intricacies of optimizing for RankBrain, we’ll delve into specific tactics and considerations for content creation, technical SEO, and ongoing measurement of performance in this dynamic digital landscape.
4. Optimizing for RankBrain
Content Relevance and Quality
Comprehensive Content Creation
RankBrain rewards content that comprehensively addresses user queries. Long-form content tends to perform well, with a study finding that the top-ranking results have an average word count of roughly 1,900 words.
Creating in-depth, authoritative content contributes to higher rankings by meeting RankBrain’s criteria for relevance and user satisfaction.
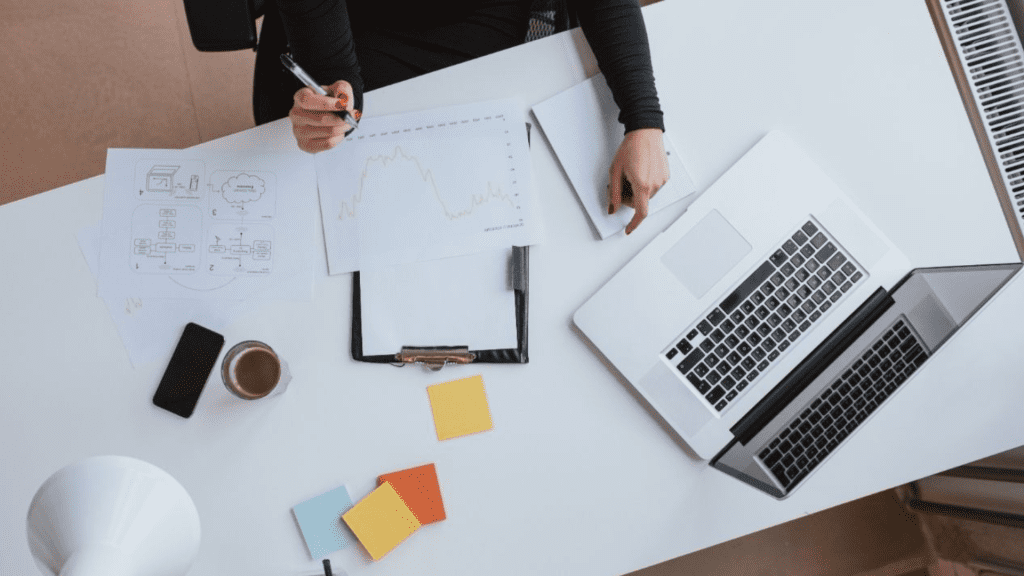
Semantic SEO and Natural Language Processing
Optimizing content for semantic relevance aligns with RankBrain’s focus on understanding context. Leveraging natural language processing and incorporating variations of key terms helps content resonate with diverse user queries.
Tools like Google’s Natural Language API can assist in analyzing and refining content to align with semantic search principles.
User Experience Signals
Dwell Time and Click-Through Rates
RankBrain places a significant emphasis on user engagement metrics.
Enhancing dwell time—measuring the duration users spend on a webpage—and click-through rates signals to RankBrain that the content is relevant and satisfying user intent.
Crafting compelling meta titles and descriptions contributes to higher click-through rates, positively influencing rankings.
Interactive and Engaging Content
The incorporation of interactive elements, such as videos, infographics, and interactive tools, enhances user engagement.
These elements contribute to a positive user experience, aligning with RankBrain’s preference for content that captivates and holds the user’s attention.
Technical SEO Considerations for RankBrain
Mobile Optimization
Given the prevalence of mobile searches, mobile optimization is imperative.
Google’s mobile-first indexing prioritizes mobile-friendly content, influencing RankBrain’s evaluation of a page’s relevance.
Tools like Google’s Mobile-Friendly Test can help assess and improve the mobile compatibility of a website.
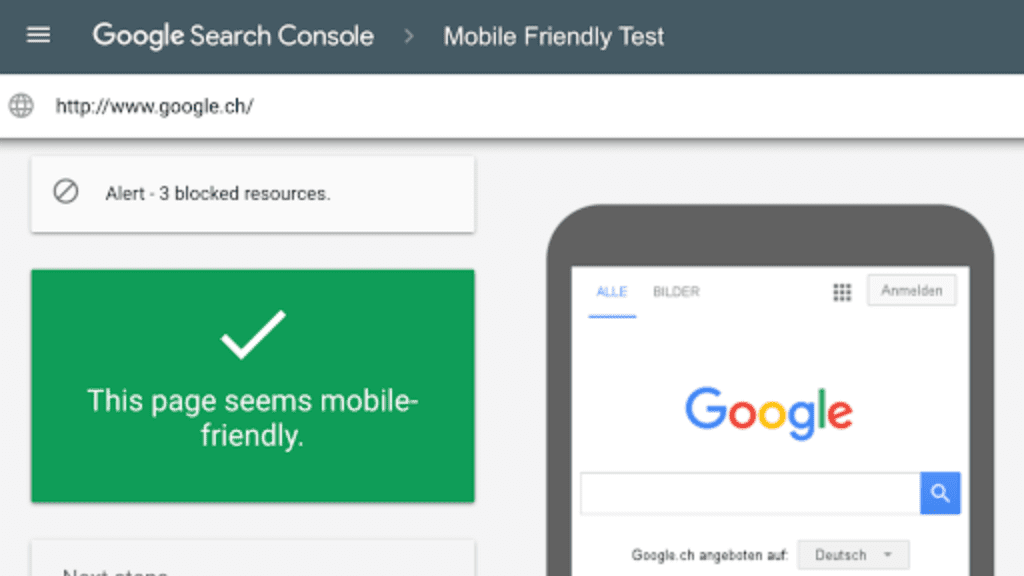
Page Speed and Core Web Vitals
Fast-loading pages contribute to a positive user experience, impacting both user satisfaction and search rankings.
Google’s Core Web Vitals, including metrics like Largest Contentful Paint and Cumulative Layout Shift, provide insights into a page’s loading performance.
Optimizing for these factors aligns with RankBrain’s preference for content that provides a seamless user experience.
Examples
Moz’s User Intent Optimization
Moz, a prominent SEO tool provider, exemplifies successful RankBrain optimization through its focus on user intent.
By aligning content with user queries and intent, Moz has established itself as a go-to resource in the SEO community.
Their blog posts often feature comprehensive guides that not only address specific topics but also anticipate related queries, aligning with RankBrain’s contextual understanding.
The Guardian’s Multimedia Integration
News outlets, such as The Guardian, understand the importance of engaging content.
The integration of multimedia elements, including images and videos, enhances user engagement and aligns with RankBrain’s preference for diverse and interactive content.
The Guardian’s adoption of multimedia elements contributes to a richer user experience and potentially higher rankings.
Google found that as page load time goes from one second to 10 seconds, the probability of a mobile site visitor bouncing increases by 123%, as cited by Search Engine Land.
This statistic underscores the critical role of page speed in retaining user attention and positively influencing RankBrain’s evaluation of a webpage.

Content-Length and Rankings
Longer content has more backlinks, which helps with ratings. Google’s ranking system prefers content on the longer side.
This data emphasizes the importance of creating comprehensive, information-rich content for optimal RankBrain optimization.
In optimizing for RankBrain, a multifaceted approach is necessary, encompassing content creation, user experience signals, and technical SEO considerations.
As we delve into measuring and tracking RankBrain performance, we’ll explore the tools and metrics essential for gauging the impact of optimization efforts in the ever-evolving landscape of search engine rankings.
5. Future Trends in Machine Learning and SEO
Advancements in Search Engine Algorithms
Evolution of Machine Learning in Search
As machine learning continues to redefine the SEO landscape, future trends in search engine algorithms are poised to be increasingly dynamic.
Google’s commitment to refining algorithms like RankBrain indicates a trajectory toward even more sophisticated machine learning integration.
Neural Matching and Semantic Understanding
Advancements in neural matching technology are enhancing search engines’ ability to understand the nuances of language.
Google’s BERT (Bidirectional Encoder Representations from Transformers) update, for instance, introduced a more context-aware understanding of words in queries, significantly impacting search results.
Predictions for the Future of RankBrain
Enhanced User Intent Recognition
Future iterations of RankBrain are likely to exhibit enhanced capabilities in recognizing and interpreting user intent.
This evolution is crucial for delivering search results that align not only with the explicit terms of a query but also with the underlying context and intent behind it.
Improved Contextual Relevance
The future of RankBrain may involve more nuanced contextual understanding.
As the algorithm evolves, it may better grasp the broader context of content, discerning relationships between topics and providing more accurate and contextually relevant search results.
Integration of AI and SEO Strategies
Conversational AI and Voice Search
The rise of conversational AI and voice search is set to reshape how users interact with search engines.
As voice-activated devices become increasingly prevalent, SEO strategies will need to adapt to the conversational nature of spoken queries.
Optimizing for featured snippets becomes crucial as they often serve as the source for voice search responses.
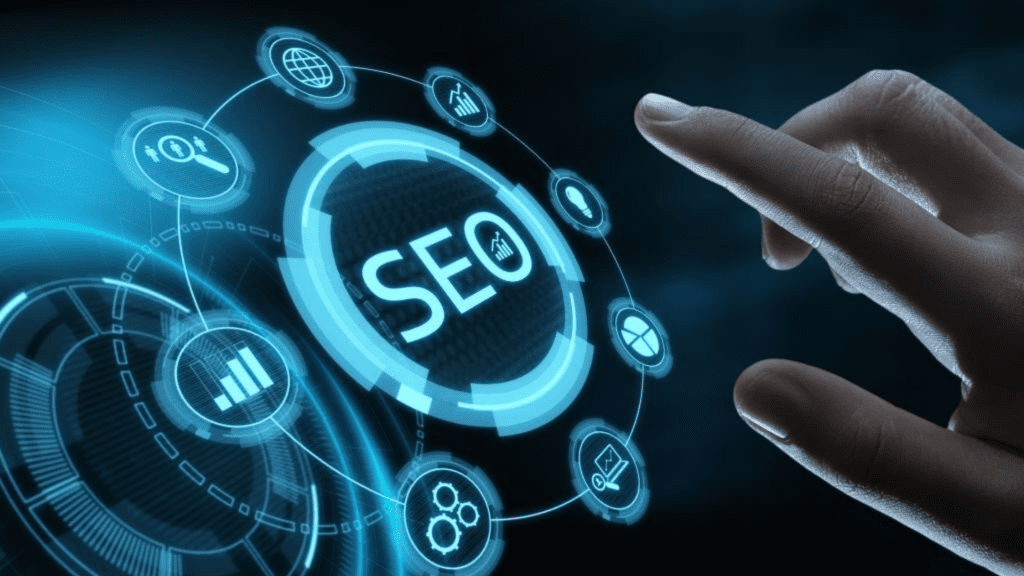
AI-Generated Content
The integration of AI in content creation is on the horizon. AI tools like OpenAI’s GPT-4 have the potential to automate the generation of high-quality content, providing valuable assistance to content creators.
However, maintaining a balance between AI-generated content and human creativity will be essential to ensure authenticity and relevance.
Machine Learning and Personalization
Hyper-Personalization in Search Results
The future of machine learning in SEO includes hyper-personalization, where search results are tailored not only to general user behaviour but also to individual preferences and historical interactions.
This level of personalization aims to deliver a highly individualized and satisfying search experience.
Predictive Search Suggestions
Anticipatory search suggestions powered by machine learning algorithms will become more prevalent. Search engines will increasingly predict user queries based on past behaviour, making the search process more efficient and user-friendly.
Voice Search Adoption
According to a survey by Adobe, 48% of consumers use voice assistants for general web searches, as cited by Search Engine Land.
As voice search continues to gain traction, the integration of conversational AI in SEO strategies becomes pivotal for staying ahead in the evolving search landscape.
AI in Content Creation
60% of marketers say generative AI will transform their role, but worry about accuracy. However, in forecasting the future trends in machine learning and SEO, the industry is poised for significant advancements.
As AI tools become more sophisticated, the potential for automating certain aspects of content creation will likely grow, revolutionizing the way content is produced for SEO purposes.
From the evolution of search engine algorithms to the integration of AI in content creation and the rise of hyper-personalization, staying abreast of these developments is essential for businesses and marketers aiming to maintain a competitive edge in the digital landscape.
Conclusion
In the ever-evolving landscape of search engine optimization, Google’s RankBrain stands as a formidable force, ushering in a new era defined by machine learning and adaptive algorithms.
Embracing the intricacies of RankBrain is not just a choice but a necessity for businesses and marketers striving to maintain a competitive edge.
The Evolution of Search Algorithms
RankBrain marks a pivotal shift from static algorithms to dynamic, self-learning systems. It signifies Google’s commitment to enhancing the search experience by harnessing the power of machine learning.
Understanding this evolution is paramount for crafting SEO strategies that resonate with the preferences of modern search engines.
RankBrain’s Crucial Role in Search
Our journey through the basics of RankBrain has unravelled its core purpose: to handle ambiguous or novel queries that traditional algorithms find challenging.
By delving into the nuances of dynamic query interpretation and adapting to user behaviour, RankBrain emerges as a linchpin in the intricate machinery of Google’s search algorithm.
Impact on Search Results and User Experience
From contextual understanding and improved ambiguity handling to enhanced personalization and localization, RankBrain is a driving force behind the delivery of more relevant and user-centric search results.
Navigating the Mechanics of RankBrain
As we navigated the mechanics of RankBrain, exploring neural networks, continuous learning iterations, and the factors considered in ranking, a deeper comprehension emerged.
RankBrain’s ability to decipher ambiguous queries and adapt to evolving search trends showcases its prowess in understanding the intricacies of user intent.
Adapting SEO Strategies for RankBrain
The section on adapting SEO strategies for RankBrain sheds light on the paradigm shift required in the optimization approach.
From content creation emphasizing semantic relevance and user engagement metrics to technical considerations like mobile optimization and page speed, a holistic strategy is essential for success in the RankBrain era.
The Future Trends in Machine Learning and SEO
Our exploration into future trends uncovered the trajectory of search engine algorithms, the predicted evolution of RankBrain, and the integration of AI in SEO strategies.
As we anticipate enhanced user intent recognition, improved contextual relevance, and the rise of conversational AI, it becomes evident that the future of SEO is intricately intertwined with machine learning advancements.
In conclusion, “Google’s RankBrain Algorithm: Understanding Machine Learning in SEO” transcends a mere exploration; it is a roadmap for navigating the ever-changing SEO terrain.
As we stand at the intersection of machine learning and search optimization, the lessons learned from RankBrain extend beyond algorithmic intricacies—they encapsulate a dynamic philosophy of user-centricity, adaptability, and continual learning.
Embrace the principles of RankBrain, cultivate an unwavering commitment to user satisfaction, and evolve your strategies in tandem with the ever-advancing landscape of machine learning.
In doing so, you not only optimize for search engines but pave the way for a seamless, relevant, and enriching online experience for your audience—a journey that aligns perfectly with the essence of Google’s RankBrain.
If you are looking for a top-class digital marketer, then book a free consultation slot here.
If you find this article useful, why not share it with your friends and business partners, and also leave a nice comment below?
We, at the AppLabx Research Team, strive to bring the latest and most meaningful data, guides, and statistics to your doorstep.
To get access to top-quality guides, click over to the AppLabx Blog.
People also ask
What is the RankBrain algorithm in SEO?
RankBrain is a machine learning component of Google’s search algorithm. It interprets and understands user queries, especially ambiguous or novel ones, improving search results. Focusing on user intent, it refines rankings by adapting to evolving search patterns, enhancing the relevance of search results.
Is RankBrain a ranking factor in Google search?
Yes, RankBrain is a significant ranking factor in Google search. As a machine learning algorithm, it interprets user queries and refines search results by understanding intent.Its adaptive nature influences rankings, emphasizing the importance of user-centric content for SEO success.
How does Google determine the ranking of content?
Google determines content rankings through complex algorithms that consider factors like relevance, user engagement, and quality. Machine learning components like RankBrain interpret user intent, while traditional factors such as keywords, backlinks, and page structure also influence rankings.