Key Takeaways
- Harness Strategic Insights: Explore applications, tools, and future trends to unlock the strategic potential of sentiment analysis in social media.
- Transform Customer Engagement: From brand monitoring to crisis management, learn how sentiment analysis elevates customer engagement and shapes brand perceptions.
- Navigate Future Trends: Stay ahead in the dynamic landscape of social media with a guide to emerging trends, including NLP advancements, AI integration, and privacy-preserving techniques.
Welcome to our comprehensive guide on “What Is Sentiment Analysis In Social Media.”
In today’s digital age, social media has become an integral part of our daily lives, serving as a platform for communication, information sharing, and expression.
With the immense volume of data generated on social media platforms every second, understanding the sentiments expressed by users has never been more crucial for businesses, brands, and individuals alike.
Sentiment analysis, also known as opinion mining, is the process of leveraging natural language processing (NLP) and machine learning techniques to analyze and determine the sentiments expressed in textual data.
In the realm of social media, where opinions are abundant and diverse, sentiment analysis emerges as a powerful tool that goes beyond mere data analysis.
It allows us to tap into the collective pulse of the online community, unveiling insights that can shape strategies, enhance customer experiences, and fortify brand reputations.
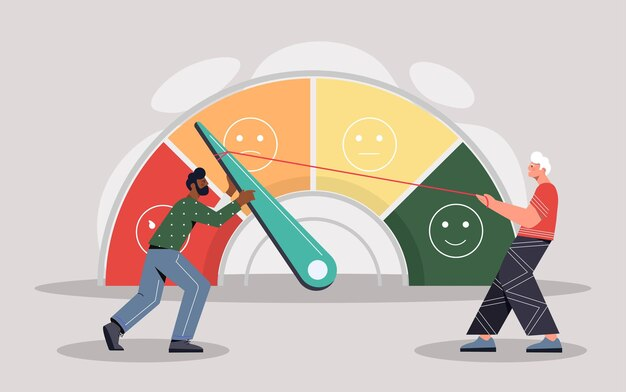
The Significance of Sentiment Analysis in Social Media
In the first section of our guide, we’ll delve into the very essence of sentiment analysis and its paramount importance in the social media landscape.
As we explore the depths of this intriguing concept, we’ll unravel how sentiment analysis acts as a digital compass, guiding businesses through the dynamic currents of public opinion.
Whether it’s gauging customer satisfaction, managing brand perception, or navigating through crises, sentiment analysis stands as a beacon of insights, illuminating the path to informed decision-making.
Applications Across the Social Media Spectrum
Sentiment analysis isn’t confined to a single niche; its applications span a broad spectrum within the social media landscape.
Whether it’s monitoring brand sentiment, analyzing customer feedback, or strategically positioning a brand amidst market chatter, we’ll uncover the diverse applications that make sentiment analysis an indispensable tool for businesses aiming to thrive in the digital realm.
The Benefits – A Competitive Edge
The next section of our guide will shine a spotlight on the myriad benefits that businesses and individuals can gain from incorporating sentiment analysis into their strategies.
Improved customer engagement, enhanced brand reputation, and real-time decision-making are just a few of the advantages that await those who harness the power of sentiment analysis.
As we delve into these benefits, you’ll discover how sentiment analysis can provide a competitive edge in an ever-evolving digital landscape.
A Step-by-Step Guide to Implementation
This section will guide you through the practical steps of implementing sentiment analysis.
From data collection and preprocessing to model selection and evaluation metrics, we’ll outline a roadmap for successfully incorporating sentiment analysis into your social media strategy.
Tools of the Trade
In the next section, we’ll explore the landscape of sentiment analysis tools and platforms.
A comparative analysis of popular tools, their features, and selection criteria will equip you with the knowledge needed to choose the right tools for your specific needs.
Peering into the Future
The penultimate section of our guide will cast a visionary gaze into the future of sentiment analysis.
From advancements in natural language processing to the integration of artificial intelligence and machine learning, we’ll explore the emerging trends that promise to shape the landscape of sentiment analysis in the years to come.
But, before we venture further, we like to share who we are and what we do.
About AppLabx
From developing a solid marketing plan to creating compelling content, optimizing for search engines, leveraging social media, and utilizing paid advertising, AppLabx offers a comprehensive suite of digital marketing services designed to drive growth and profitability for your business.
AppLabx is well known for helping companies and startups use social media to drive web traffic to their websites and web apps.
At AppLabx, we understand that no two businesses are alike. That’s why we take a personalized approach to every project, working closely with our clients to understand their unique needs and goals, and developing customized strategies to help them achieve success.
If you need a digital consultation, then send in an inquiry here.
What Is Sentiment Analysis In Social Media: A Complete Guide
- How Sentiment Analysis Works
- Applications of Sentiment Analysis in Social Media
- Benefits of Implementing Sentiment Analysis
- Steps to Perform Sentiment Analysis
- Popular Sentiment Analysis Tools and Platforms
- Future Trends in Sentiment Analysis
1. How Sentiment Analysis Works
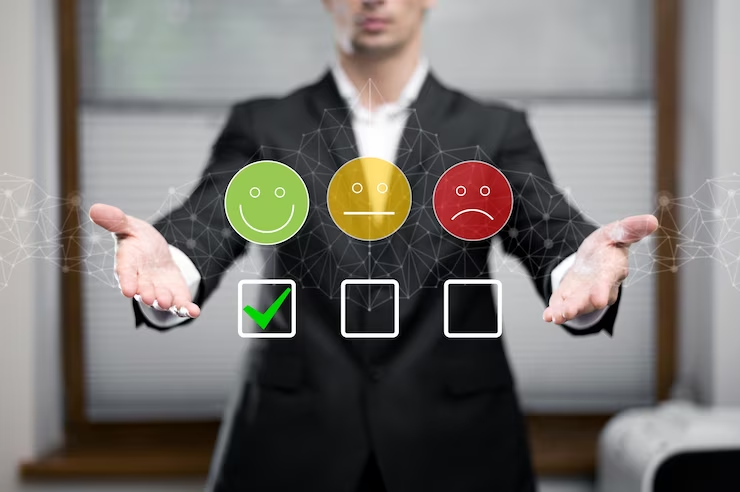
The Mechanism Behind Sentiment Analysis
Sentiment analysis, also known as opinion mining, operates at the intersection of natural language processing (NLP) and machine learning.
In this section, we’ll dissect the intricate mechanism that powers sentiment analysis and explore the technologies and techniques that enable it to discern sentiments within vast amounts of textual data.
Natural Language Processing (NLP): The Foundation of Sentiment Analysis
Sentiment analysis relies heavily on Natural Language Processing (NLP) to understand and interpret human language.
NLP algorithms are designed to recognize patterns, relationships, and meanings within textual data, allowing computers to comprehend the nuances of human communication.
The key components of NLP in sentiment analysis include:
- Tokenization: Breaking down text into individual words or phrases, known as tokens.
- Part-of-Speech Tagging: Assigning grammatical categories (nouns, verbs, adjectives) to tokens.
- Named Entity Recognition (NER): Identifying entities such as names, locations, and organizations.
- Syntax Analysis: Analyzing the grammatical structure of sentences.
Example: Consider the sentence “I love this product, but the customer service needs improvement.”
NLP helps break down this sentence into tokens, recognize the sentiment-bearing words (“love” and “needs improvement”), and understand the overall sentiment as a mix of positive and negative.
Machine Learning Models in Sentiment Analysis
Machine learning models play a pivotal role in sentiment analysis by learning from labelled datasets and making predictions on unseen data. There are two main types of sentiment analysis models:
- Supervised Learning: These models are trained on labelled datasets where each piece of text is associated with a sentiment label (positive, negative, or neutral).
Common supervised learning algorithms include Support Vector Machines (SVM), Naive Bayes, and deep learning models like Long Short-Term Memory (LSTM) networks. - Unsupervised Learning: These models operate without labelled data and aim to discover patterns and sentiments autonomously. Techniques such as clustering and topic modelling fall under unsupervised learning.
Example: A supervised learning model trained on a dataset of customer reviews can learn to associate specific words or phrases with positive or negative sentiments.
For instance, the model might learn that words like “excellent,” “pleased,” and “responsive” are indicative of positive sentiments.
Sentiment Lexicons and Dictionaries
Sentiment lexicons and dictionaries serve as reference resources containing words and their associated sentiments.
These pre-built dictionaries help sentiment analysis models by providing a basis for understanding the sentiment polarity of words.
Lexicons often assign a score or label to words, indicating whether they convey a positive, negative, or neutral sentiment.
Example: If a user’s social media post contains the word “amazing,” the sentiment lexicon would provide the model with information that “amazing” is a positive sentiment word.
Contextual Understanding and Semantic Analysis
Sentiment analysis goes beyond the mere identification of positive or negative words; it involves understanding the context and nuances of language.
Semantic analysis helps discern the deeper meaning of phrases, considering the relationships between words and the overall context of the text.
Example: In the sentence “The weather is so hot, it’s cool,” semantic analysis is crucial for recognizing that, despite the word “hot,” the sentiment is positive, as the context implies the weather is enjoyable.
Handling Negations and Modifiers
Sentiment analysis must grapple with linguistic constructs such as negations and modifiers, which can significantly alter the sentiment expressed.
Advanced models take into account the impact of words like “not” and modifiers that intensify or diminish sentiments.
Example: In the phrase “The product is not bad,” recognizing the negation is vital for understanding that, despite the negative word “bad,” the overall sentiment is positive.
The Integration of Sentiment Analysis Technologies
As technology continues to evolve, sentiment analysis is becoming more sophisticated, incorporating advancements in deep learning, reinforcement learning, and ensemble methods.
The integration of these technologies enhances the accuracy and efficiency of sentiment analysis models, making them more adept at capturing the intricacies of human emotions in textual data.
Deep Learning in Sentiment Analysis
Deep learning, particularly neural networks, has revolutionized sentiment analysis.
These models, such as recurrent neural networks (RNNs) and transformer-based architectures like BERT (Bidirectional Encoder Representations from Transformers), excel in capturing complex relationships within language, enabling them to discern subtle sentiments.
Example: BERT, with its bidirectional context understanding, can grasp the meaning of words in relation to the entire sentence, addressing the limitations of traditional models that process text in a unidirectional manner.
Reinforcement Learning for Sentiment Analysis
Reinforcement learning techniques are being explored to enhance sentiment analysis models by allowing them to learn and adapt based on feedback.
These models can improve over time, refining their understanding of sentiments through a continuous feedback loop.
Example: A sentiment analysis system utilizing reinforcement learning could receive feedback on its predictions and adjust its model parameters to improve accuracy in future analyses.
Ensemble Methods for Enhanced Accuracy
Ensemble methods involve combining predictions from multiple models to achieve better overall accuracy. In sentiment analysis, combining the outputs of different models or leveraging diverse feature sets can lead to more robust and reliable sentiment predictions.
Example: An ensemble approach might involve combining the predictions of a deep learning model, a traditional machine learning model, and a lexicon-based model to create a more comprehensive sentiment analysis system.
2. Applications of Sentiment Analysis in Social Media
Sentiment analysis is a versatile tool with applications that span the entire spectrum of social media interactions.
In this section, we’ll explore how businesses, brands, and individuals leverage sentiment analysis to gain valuable insights, enhance customer experiences, and shape their digital strategies.
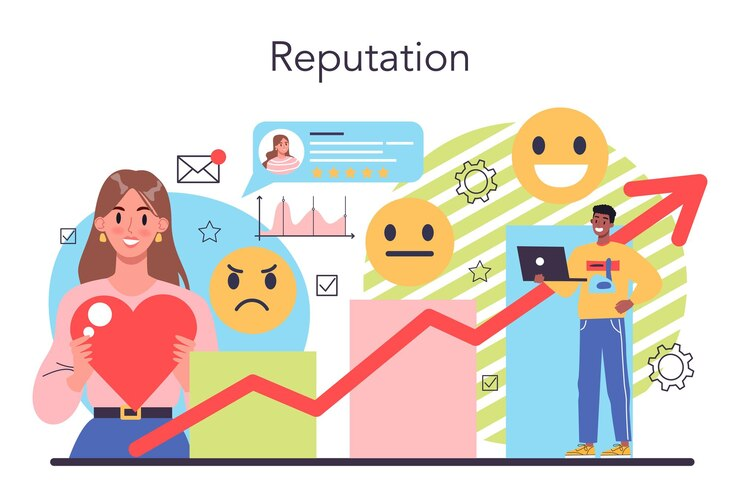
Brand Monitoring and Reputation Management
Sentiment analysis serves as a vigilant guardian for brands seeking to monitor their online presence and manage their reputation effectively.
By analyzing social media mentions, reviews, and comments, businesses can gauge public sentiment toward their brand.
- Example: A major consumer electronics brand utilizes sentiment analysis to track mentions on various social platforms.
By identifying positive sentiments, they can amplify successful marketing campaigns, while addressing negative sentiments promptly helps in damage control. - According to a report, 91% of 18 to 34-year-olds trust reviews online just as much as personal recommendations, highlighting the critical role sentiment analysis plays in brand perception.
Customer Feedback Analysis for Product Improvement
Social media platforms serve as a treasure trove of customer feedback. Sentiment analysis enables businesses to extract valuable insights from this feedback, identifying areas of improvement, and enhancing their products or services based on customer preferences.
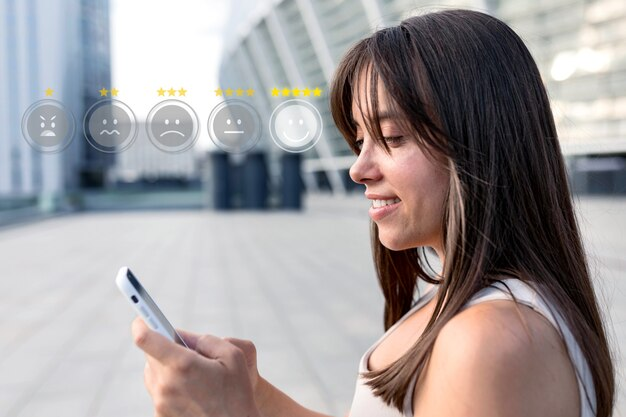
Example: An e-commerce platform utilizes sentiment analysis on customer reviews to identify common issues with a product.
The analysis reveals recurring negative sentiments related to a specific feature, prompting the company to address the issue in the next product iteration.
Crisis Management and Proactive Communication
Sentiment analysis acts as an early warning system for potential crises brewing on social media.
By monitoring sentiment during sensitive situations, businesses can detect issues before they escalate, allowing for proactive crisis management and strategic communication.
Example: A global airline employs sentiment analysis during times of unexpected flight disruptions. By monitoring social media sentiment, the airline can quickly identify frustrated passengers and address concerns in real-time, mitigating reputational damage.
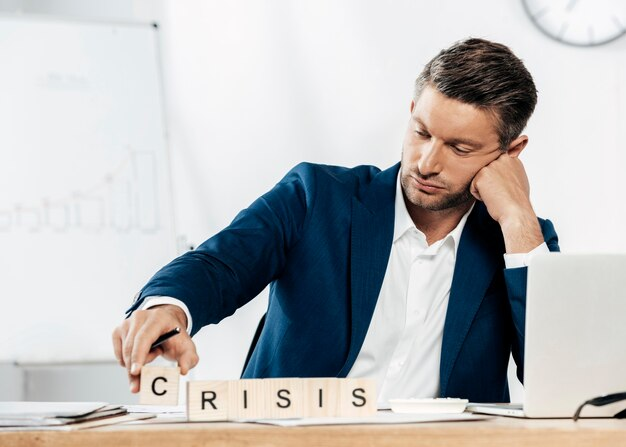
Market Research and Competitor Analysis
Social media is a goldmine for market researchers looking to understand consumer preferences, trends, and competitive landscapes. Sentiment analysis aids in extracting actionable insights from vast datasets, guiding businesses in strategic decision-making.
Example: A fashion retailer uses sentiment analysis to analyze social media discussions around fashion trends.
By identifying sentiments associated with specific styles, colors, or designers, the retailer gains valuable market intelligence to optimize inventory and marketing strategies.
Social Listening for Customer Engagement
Social media is not just a platform for broadcasting messages; it’s also a channel for meaningful conversations. Sentiment analysis enables businesses to practice social listening, engage with customers in real-time, and foster a sense of community.
- Example: A hospitality brand actively monitors social media sentiments related to customer experiences.
Positive sentiments trigger personalized thank-you messages, while negative sentiments prompt the brand to reach out, demonstrating a commitment to customer satisfaction. - A survey found that nearly 70% expect a response within 24 hours or less, underscoring the importance of timely and personalized engagement facilitated by sentiment analysis.
Campaign Effectiveness Measurement
For marketers, evaluating the success of social media campaigns is paramount. Sentiment analysis provides a quantitative measure of campaign effectiveness by analyzing the sentiments expressed in response to marketing initiatives.
Example: An e-commerce company launches a new advertising campaign and uses sentiment analysis to measure the reactions on social media. Positive sentiments indicate a successful campaign, while negative sentiments prompt a reevaluation of messaging.
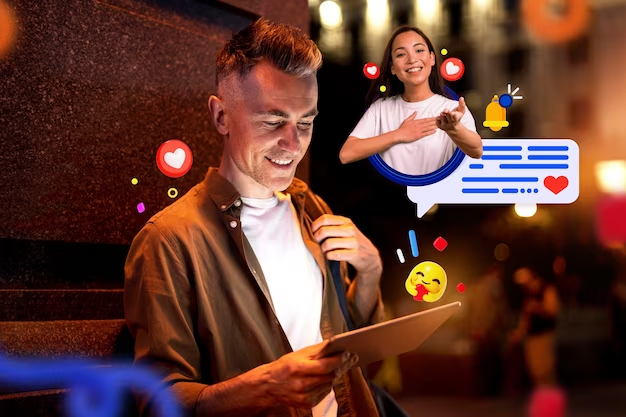
3. Benefits of Implementing Sentiment Analysis
Sentiment analysis is more than just a technological marvel; it’s a strategic asset that can redefine how businesses engage with their audience, enhance decision-making processes, and fortify their brand presence.
In this section, we’ll delve into the manifold benefits of implementing sentiment analysis.
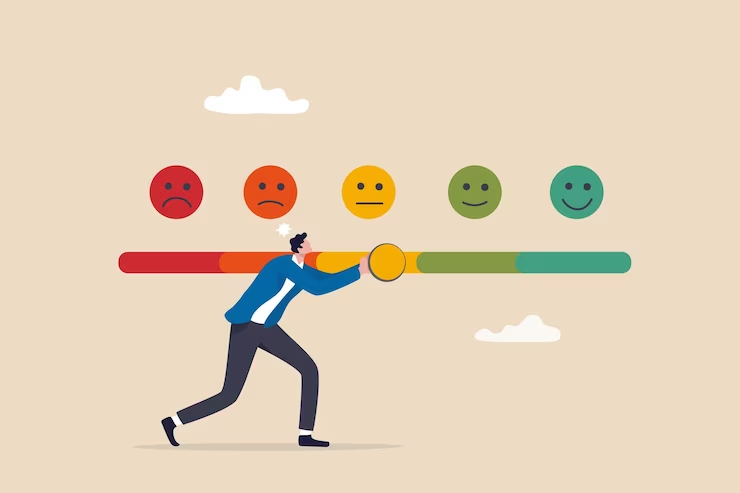
Improved Customer Engagement and Relationship Building
Sentiment analysis acts as a bridge between businesses and their customers, enabling proactive engagement based on real-time insights into customer sentiments.
- Example: A leading online retailer employs sentiment analysis to understand customer sentiments during promotional campaigns.
By identifying positive sentiments, the retailer tailors personalized thank-you messages and exclusive offers, fostering a stronger connection with customers. - According to a report, 71 per cent of consumers expect companies to deliver personalized interactions, emphasizing the importance of personalized engagement facilitated by sentiment analysis.
Enhanced Brand Reputation Management
Maintaining a positive brand image is paramount in the digital age.
Sentiment analysis serves as a vigilant guardian, allowing businesses to detect and address negative sentiments promptly, safeguarding their brand reputation.
Example: A multinational tech company monitors social media sentiments to identify potential PR crises. If negative sentiments arise, the company swiftly responds with transparent communication, showcasing a commitment to resolving issues and maintaining trust.
Real-Time Decision Making in Marketing Strategies
The rapid pace of social media requires businesses to make swift decisions. Sentiment analysis provides real-time insights, allowing marketers to adapt and optimize strategies based on the current sentiments of their target audience.
- Example: An automotive company uses sentiment analysis during a product launch to gauge initial customer reactions.
Positive sentiments guide the company to focus marketing efforts on praised features while addressing negative sentiments informs immediate adjustments to messaging. - A study revealed that 59% of consumers prefer to buy products from familiar brands, highlighting the importance of aligning marketing strategies with positive sentiments.
Competitive Advantage Through Market Intelligence
Sentiment analysis goes beyond analyzing individual brand mentions; it offers a panoramic view of market trends, consumer preferences, and competitor sentiments, providing businesses with a competitive edge.
- Example: A beverage company monitors social media sentiments not only for its own brand but also for competitors.
By identifying gaps in competitor offerings or understanding customer preferences, the company gains a strategic advantage in product development and marketing. - According to a survey, executive teams that make extensive use of customer data analytics across all business decisions see a 126% profit improvement over companies that don’t.
Tailored Product Development and Innovation
Understanding customer sentiments allows businesses to align their product development strategies with consumer preferences, leading to more successful innovations and a higher likelihood of meeting market demands.
Example: A tech startup leverages sentiment analysis on user feedback to refine its software. Positive sentiments guide the enhancement of well-received features while addressing negative sentiments helps in identifying and rectifying software bugs promptly.
Optimized Customer Support and Issue Resolution
Sentiment analysis plays a pivotal role in transforming customer support by identifying issues in real-time, enabling businesses to provide swift resolutions and enhance overall customer satisfaction.
Example: An e-commerce platform utilizes sentiment analysis on customer reviews to identify common issues. Negative sentiments trigger automated alerts, prompting customer support to proactively reach out to affected customers and resolve their concerns.
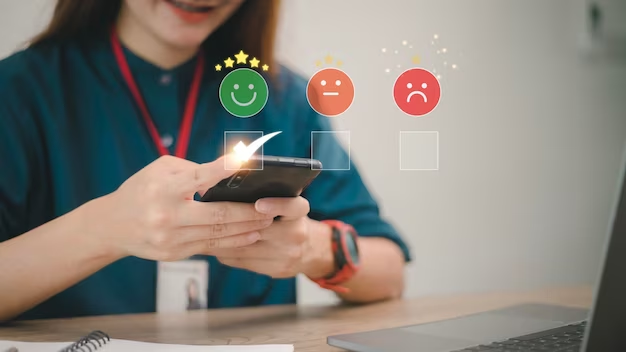
Quantifiable Measurement of Marketing ROI
Implementing sentiment analysis provides businesses with a tangible means of measuring the return on investment (ROI) of their marketing efforts. By quantifying the sentiments generated by marketing campaigns, businesses can gauge their impact on customer perceptions.
Example: A digital marketing agency utilizes sentiment analysis metrics to assess the success of social media campaigns. Positive sentiments, increased brand mentions, and improved sentiment scores serve as indicators of a campaign’s effectiveness.
4. Steps to Perform Sentiment Analysis
Sentiment analysis is a nuanced process that involves several crucial steps, from collecting relevant data to selecting appropriate models for analysis.
In this comprehensive guide, we will walk through each step of performing sentiment analysis.
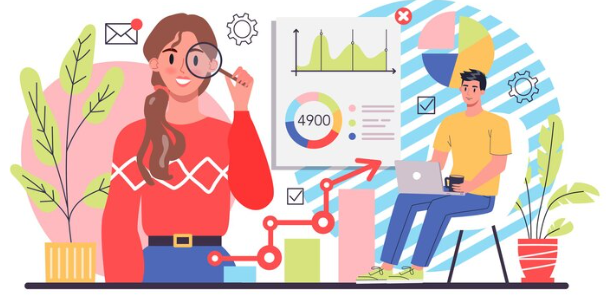
Data Collection: Harvesting the Seeds of Sentiment
The first step in sentiment analysis is to gather a diverse and representative dataset that accurately reflects the sentiments you aim to analyze. Data sources can include social media posts, customer reviews, survey responses, and other text-based interactions.
Example: A mobile app developer collects user reviews from app stores and social media platforms to analyze sentiments about their app’s features, usability, and overall user experience.
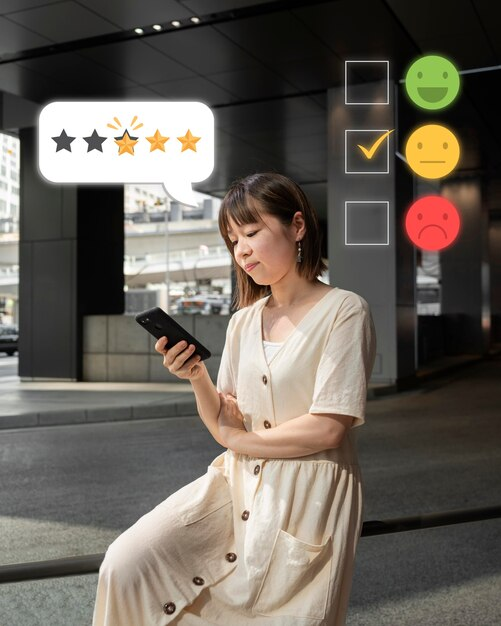
Data Preprocessing: Cleaning the Canvas for Analysis
Raw text data often contains noise, including irrelevant information, emojis, and special characters. Data preprocessing involves cleaning and formatting the text to enhance the accuracy of sentiment analysis models.
- Example: A restaurant chain conducting sentiment analysis on customer feedback removes irrelevant information such as timestamps and location tags from social media comments to focus on the core sentiment.
- Data scientists spend 60% of their time cleaning and organizing data, underscoring the importance of data preprocessing in any data analysis task.
Model Selection: Choosing the Right Tool for the Job
There are various sentiment analysis models available, each with its strengths and weaknesses. The choice of model depends on factors such as the complexity of the task, available resources, and the nature of the data.
- Example: An e-commerce platform may opt for a pre-trained deep learning model like BERT for sentiment analysis due to its ability to capture contextual nuances in customer reviews and comments.
- A study by McKinsey found that companies with a proactive AI strategy achieve an operating profit margin about seven percentage points above the industry average.
Training and Testing: Nurturing the Analytical Mindset
Before deploying a sentiment analysis model, it must be trained on a labelled dataset. This involves providing the model with examples of text and their corresponding sentiments. Subsequently, the model’s performance is tested on a separate dataset to ensure its accuracy.
- Example: A movie review website trains a sentiment analysis model on a dataset of movie reviews labelled as positive or negative. The model is then tested on new reviews to assess its ability to accurately predict sentiments.
- According to a report, the global machine-learning market is expected to reach USD 117.19 billion by 2027, reflecting the growing adoption of machine-learning technologies across various industries.
Evaluation Metrics: Assessing the Analytical Aptitude
To measure the effectiveness of a sentiment analysis model, various evaluation metrics are employed, such as accuracy, precision, recall, and F1 score. These metrics provide insights into how well the model performs in distinguishing between different sentiment classes.
Example: An airline uses precision as a key metric to evaluate its sentiment analysis model. High precision ensures that when the model predicts a negative sentiment, it is accurate and trustworthy.
5. Popular Sentiment Analysis Tools and Platforms
Sentiment analysis has witnessed a surge in popularity, leading to the development of diverse tools and platforms designed to simplify and enhance the sentiment analysis process.
In this section, we’ll delve into some of the widely used sentiment analysis tools and platforms, shedding light on their features, functionalities, and how businesses can leverage them for actionable insights.
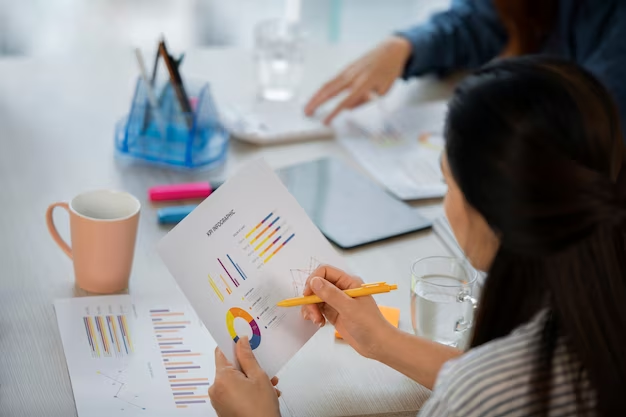
Natural Language Processing (NLP) Libraries
NLP libraries provide a foundational framework for sentiment analysis, offering a suite of tools and functionalities that streamline text-processing tasks.
- NLTK (Natural Language Toolkit): NLTK, a Python library, is renowned for its robust set of tools for tasks such as tokenization, stemming, and part-of-speech tagging.
- spaCy: spaCy is a Python library known for its efficiency in linguistic annotation and dependency parsing, making it a valuable asset for sentiment analysis tasks.
- Example: A social media analytics firm utilizes NLTK for initial text preprocessing, tokenizing, and cleaning social media data before passing it to a sentiment analysis model.

Machine Learning Frameworks for Sentiment Analysis
Machine learning frameworks offer a more advanced approach to sentiment analysis, enabling businesses to train custom models on specific datasets.
- TensorFlow: TensorFlow, an open-source machine learning framework, provides tools for building and training sentiment analysis models, including neural networks.
- Scikit-Learn: Scikit-Learn, a versatile machine learning library, offers algorithms and tools for tasks such as text classification, a fundamental component of sentiment analysis.
- Example: An e-commerce giant utilizes TensorFlow to develop a custom sentiment analysis model trained on customer reviews, enabling more accurate sentiment predictions tailored to their domain.

Pre-built Sentiment Analysis APIs
For businesses seeking quick integration without extensive model development, pre-built sentiment analysis APIs offer a convenient solution.
- Google Cloud Natural Language API: Google’s API provides sentiment analysis capabilities, offering pre-trained models for extracting sentiments from text.
- IBM Watson Natural Language Understanding: IBM’s API includes sentiment analysis features that businesses can integrate into applications to gain insights into user sentiments.
- Example: A marketing analytics firm incorporates Google Cloud Natural Language API to analyze sentiments in social media data, enabling clients to gauge public reactions to marketing campaigns.
Social Media Listening Tools
Social media listening tools are specifically designed to monitor and analyze sentiments expressed on various social media platforms.
- Hootsuite: Hootsuite’s sentiment analysis feature allows businesses to track mentions and sentiments about their brand on social media, providing real-time insights.
- Brandwatch: Brandwatch is a social listening tool equipped with sentiment analysis capabilities, enabling users to understand public opinions and trends.
- Example: A global consumer goods company employs Hootsuite to track sentiment trends on social media, enabling it to adapt marketing strategies based on real-time consumer feedback.
- According to a report by Statista, the global social media analytics market is projected to surpass 26 billion dollars in 2028, underlining the growing significance of tools in this category.
Lexicon-Based Sentiment Analysis Tools
Lexicon-based tools rely on predefined dictionaries or word lists to determine sentiment polarity, making them suitable for scenarios where machine-learning models may not be feasible.
- VADER (Valence Aware Dictionary and sEntiment Reasoner): VADER is a lexicon-based sentiment analysis tool known for its ability to handle social media text, including emojis and colloquial expressions.
- SentiWordNet: SentiWordNet is a lexical resource that assigns sentiment scores to words, aiding in sentiment analysis tasks.
- Example: An online forum platform integrates VADER to automatically analyze sentiments in user comments, categorizing them as positive, negative, or neutral.
6. Future Trends in Sentiment Analysis
Sentiment analysis is evolving rapidly, driven by advancements in technology and an ever-growing pool of data.
In this section, we’ll explore the future trends shaping the landscape of sentiment analysis, offering a glimpse into the innovative methodologies and technologies that will redefine how sentiments are analyzed and interpreted.
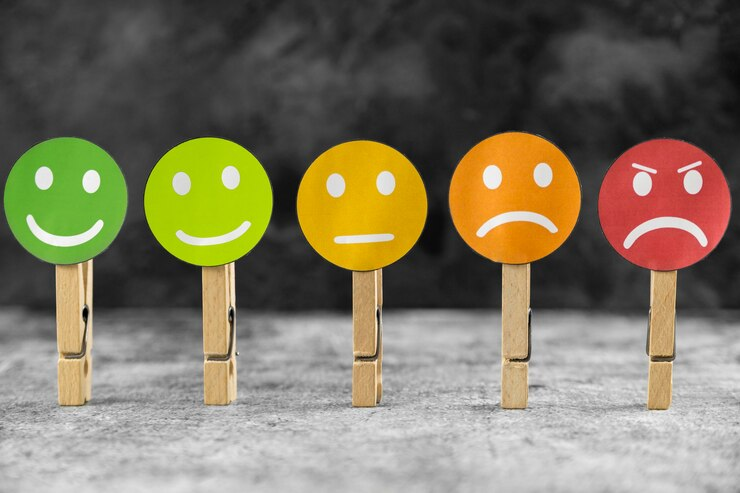
Advancements in Natural Language Processing (NLP)
As the foundation of sentiment analysis, NLP continues to evolve, with ongoing research focusing on improving contextual understanding, semantic analysis, and the handling of linguistic nuances.
- BERT (Bidirectional Encoder Representations from Transformers): BERT, a transformer-based model developed by Google, has set a benchmark for contextual understanding in NLP.
Future trends may see enhancements and adaptations of BERT-like architectures for sentiment analysis. - ELMo (Embeddings from Language Models): ELMo is another contextual embedding model that captures word meanings based on their context within a sentence, contributing to more nuanced sentiment analysis.
- Example: A social media analytics platform integrates BERT for sentiment analysis, allowing it to grasp the context of user posts and comments more effectively, leading to improved sentiment predictions.
- According to a report by MarketsandMarkets, the global natural language processing (NLP) market size is expected to reach USD 68.1 billion by 2028, indicating the growing importance and investment in NLP technologies.
Integration with Artificial Intelligence (AI) and Machine Learning (ML)
The synergy between sentiment analysis and AI/ML is expected to deepen, enabling more sophisticated models that adapt and learn from evolving linguistic patterns.
- Reinforcement Learning Models: Sentiment analysis models employing reinforcement learning techniques will become more prevalent, allowing systems to adapt and improve based on user feedback.
- Ensemble Learning Approaches: The integration of ensemble learning methods, combining predictions from multiple models, will enhance the robustness and accuracy of sentiment analysis systems.
- Example: An e-commerce platform deploys a sentiment analysis model that leverages reinforcement learning to adapt to changing customer sentiments, providing more accurate product recommendations over time.
- The global artificial intelligence market is projected to reach a revenue of $1,345.2 billion by 2030, highlighting the expansive opportunities for integrating AI in sentiment analysis.
Multimodal Sentiment Analysis
The future of sentiment analysis extends beyond text alone, embracing multimodal analysis that incorporates images, videos, and audio data for a more comprehensive understanding of sentiments.
- Image Sentiment Analysis: Techniques for analyzing sentiments expressed in images will gain prominence, allowing businesses to extract valuable insights from visual content shared on social media.
- Video Sentiment Analysis: Sentiment analysis tools will evolve to analyze sentiments expressed in video content, providing a deeper understanding of user emotions and reactions.
- Example: A marketing agency incorporates image sentiment analysis to assess the visual content shared by influencers, gaining insights into how the visual elements contribute to audience engagement.
- The image recognition market is projected to grow at a CAGR of 15.1% during the forecast period to reach USD 53.0 billion by 2025 from an estimated USD 26.2 billion in 2020, indicating the growing significance of visual data analysis.
Emotion Analysis for Deeper Insights
Future sentiment analysis models will not only determine positive or negative sentiments but also delve into nuanced emotion analysis, providing a more detailed understanding of user feelings.
- Emotion Classification Models: Sentiment analysis tools will incorporate models that classify emotions such as joy, sadness, anger, and surprise, offering a richer emotional spectrum.
- Contextual Emotional Analysis: Advances in contextual emotional analysis will enable systems to understand how emotions evolve within a conversation or over time.
- Example: A customer service chatbot incorporates emotion analysis to respond empathetically to user queries, adapting its tone and responses based on the detected emotions.
Privacy-Preserving Sentiment Analysis
With increasing concerns about data privacy, the future of sentiment analysis will witness the development of techniques that prioritize user privacy while still extracting valuable insights.
- Federated Learning Models: Sentiment analysis models employing federated learning will allow training on decentralized data sources without exposing individual user data.
- Differential Privacy Techniques: The adoption of differential privacy techniques will safeguard user identities while still enabling accurate sentiment analysis on aggregated data.
- Example: A social media platform implements federated learning for sentiment analysis, ensuring that user data remains on users’ devices and is not centralized, addressing privacy concerns.
- The privacy management software market size is expected to grow from USD 2.7 billion in 2023 to USD 15.2 billion by 2028, indicating a growing awareness of and investment in privacy-centric technologies.
Cross-Lingual and Cross-Cultural Sentiment Analysis
As businesses operate on a global scale, the future of sentiment analysis will see a focus on cross-lingual and cross-cultural analysis to ensure accurate insights across diverse linguistic and cultural contexts.
- Multilingual Models: Sentiment analysis tools will be designed to understand sentiments expressed in multiple languages, accommodating global communication.
- Cultural Sensitivity Models: Future sentiment analysis models will incorporate cultural nuances, adapting to the diverse ways sentiments are expressed across different cultures.
- Example: An international e-commerce platform employs a sentiment analysis model that supports multiple languages, allowing it to gauge customer sentiments across various regions.
Conclusion
In this comprehensive guide, we’ve embarked on a journey through the intricate world of sentiment analysis in social media, unraveling its intricacies, applications, methodologies, and future trends.
From the foundational understanding of sentiment analysis to the exploration of cutting-edge technologies, we’ve delved into the nuances that define this evolving field.
Sentiment Analysis Unveiled: A Recap
At its core, sentiment analysis is a powerful tool that empowers businesses to decipher the emotions and opinions embedded in vast volumes of social media data.
From positive endorsements to constructive criticisms, sentiment analysis extracts actionable insights that can shape marketing strategies, enhance customer experiences, and fortify brand reputations.
Applications Across Industries: Transformative Insights
We explored how sentiment analysis extends its influence across diverse industries.
From brand monitoring and customer feedback analysis to crisis management and market research, businesses are harnessing sentiment analysis to gain a competitive edge and navigate the dynamic landscape of social media with strategic acumen.
The Mechanics Behind Sentiment Analysis: A Deep Dive
Diving into the mechanics of sentiment analysis, we dissected the essential components that power this technology.
Natural Language Processing (NLP), machine learning models, sentiment lexicons, and semantic analysis collectively contribute to the nuanced understanding of textual data, enabling sentiment analysis to transcend mere identification to contextual comprehension.
Embracing Technologies and Tools: A Sentiment Analysis Toolbox
Our exploration expanded into the rich array of tools and technologies available for sentiment analysis.
From Natural Language Processing (NLP) libraries and machine learning frameworks to pre-built sentiment analysis APIs, businesses have a plethora of options to choose from based on their specific needs and resources.
Future Trends: Pioneering the Next Frontier
As we glimpsed into the future of sentiment analysis, we identified emerging trends that promise to reshape the landscape.
Advancements in Natural Language Processing, integration with AI and machine learning, multimodal analysis, emotion analysis, privacy-preserving techniques, and cross-lingual capabilities stand as beacons guiding the future trajectory of sentiment analysis.
The Road Ahead: Strategic Adoption and Continuous Innovation
In conclusion, the adoption of sentiment analysis is not merely a technological choice; it’s a strategic imperative for businesses aiming to thrive in the digital era.
As social media continues to evolve, so too must our approaches to understanding the sentiments expressed within it.
Strategic adoption of sentiment analysis tools, continuous innovation, and a proactive embrace of emerging trends will be the hallmarks of success in navigating the ever-changing landscape of social media sentiment.
If you are looking for a top-class digital marketer, then book a free consultation slot here.
If you find this article useful, why not share it with your friends and business partners, and also leave a nice comment below?
We, at the AppLabx Research Team, strive to bring the latest and most meaningful data, guides, and statistics to your doorstep.
To get access to top-quality guides, click over to the AppLabx Blog.
People also ask
What is sentiment analysis in social media?
Sentiment analysis in social media is the process of using natural language processing and machine learning to evaluate and interpret the emotions, opinions, and attitudes expressed in user-generated content. It helps businesses understand public perception, enhance brand reputation, and make data-driven decisions.
What is sentiment analysis comprehensive beginners guide?
A sentiment analysis comprehensive beginner’s guide provides an in-depth overview for newcomers, covering the basics, applications, tools, and future trends of sentiment analysis. It equips beginners with the knowledge to navigate the dynamic landscape of understanding and interpreting sentiments in textual data.
How do you explain sentiment analysis?
Sentiment analysis involves using technology to analyze and interpret emotions expressed in text data. Utilizing natural language processing and machine learning, it gauges sentiments as positive, negative, or neutral, providing valuable insights for businesses to understand public opinions, enhance customer experiences, and shape strategic decisions.